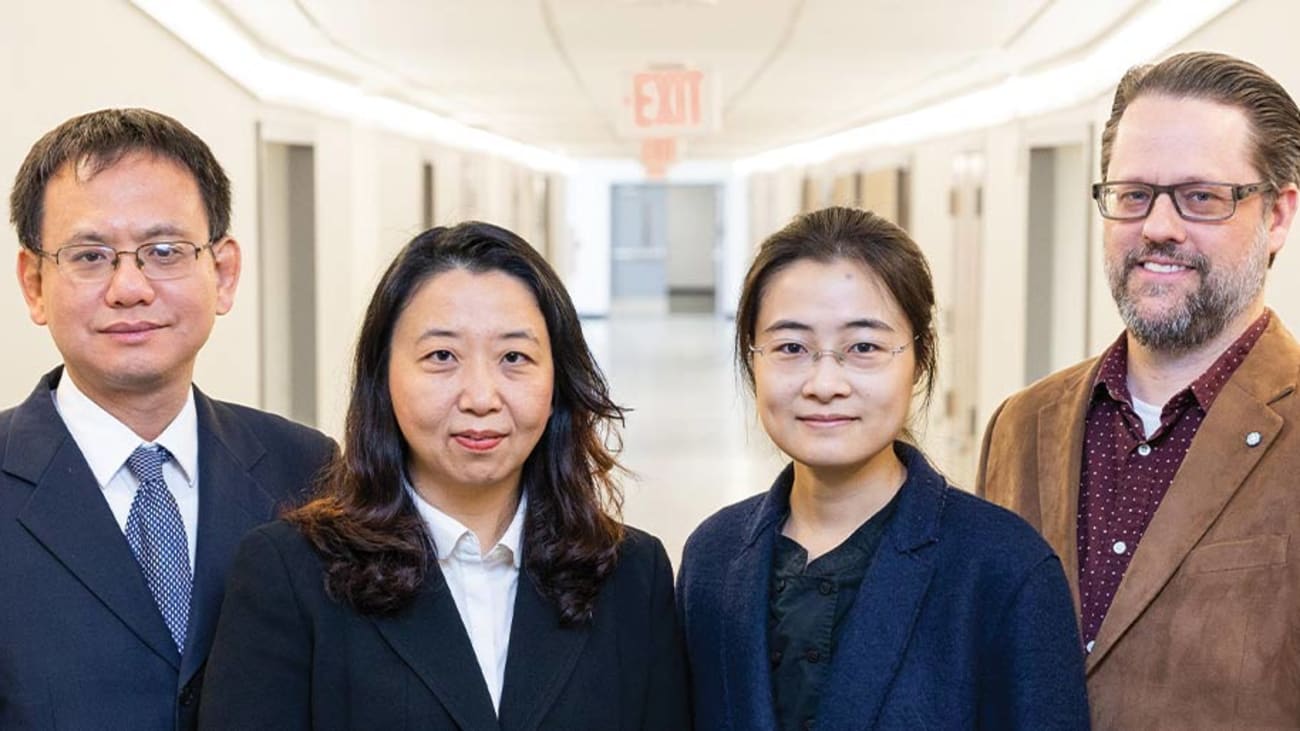
UT Southwestern research combining artificial intelligence with traditional pathology analysis holds potential for quickly creating a personalized attack plan for cancer patients when speed is essential: as non-small cell lung cancers spread. This approach identified lung cancers that are most likely to respond to one common treatment versus those that might benefit from a different approach.
“We found that combining digitized clinical pathology slides with AI algorithms holds the potential to improve precision treatment of cancer patients,” said Dr. Yang Xie, Ph.D., Professor in the Peter O'Donnell School of Public Health and Lyda Hill Department of Bioinformatics at UTSW. Dr. Xie, also Associate Dean of Data Sciences at UT Southwestern Medical School, is one of three corresponding authors on the Journal of Clinical Investigation study. The other corresponding authors are Guanghua “Andy” Xiao, Ph.D., a Professor of Bioinformatics and in the O’Donnell Jr. School of Public Health, and Mark G. Kris of Memorial Sloan Kettering Cancer Center, New York, New York.
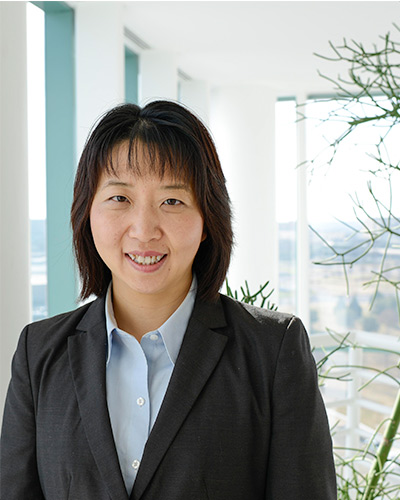
Yang Xie, Ph.D.
The investigation used pathology slides of metastatic non-small cell lung cancers from 272 patients with the epidermal growth factor receptor (EGFR) gene mutation who were enrolled in two studies conducted by the Lung Cancer Mutation Consortium, an association of more than 20 U.S. cancer centers working to match patients with targeted drugs and clinical trials for cancers of the chest area.
Dr. Xie explained that tyrosine kinase inhibitors (TKIs) – the main class of drugs targeting the EGFR pathway – are effective for many patients with tumors that contain EGFR mutations. However, some tumors – even those containing EGFR-sensitizing mutations – are unresponsive to TKIs. The goal of the study was to find a way to determine tumor characteristics that would predict responsiveness or lack thereof.
“By studying interactions between tumor cells and cells in the microenvironment that surrounds tumors, we found an important marker that could predict which metastatic cancers would show resistance to TKIs,” she said. “Tumor cells that interacted the most with nearby stromal cells were the more resistant. We think that indicates those cancers might benefit from a multipronged approach targeting multiple cell signaling pathways.”
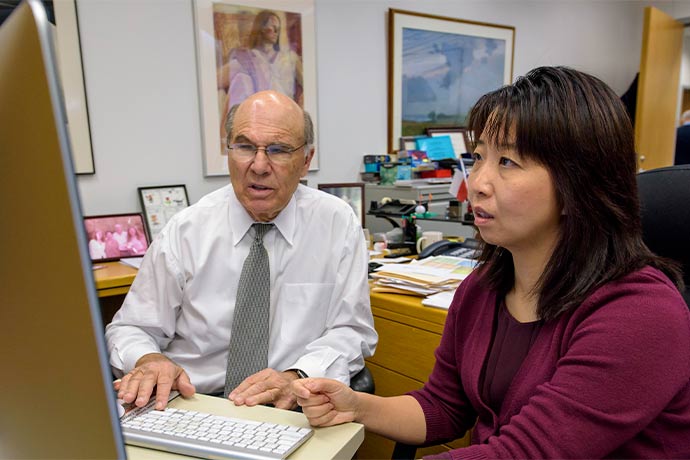
John Minna, M.D., and Yang Xie, Ph.D.
Importantly, this approach could easily be used by pathology departments across the country, added John Minna, M.D., Director of the Hamon Center for Therapeutic Oncology Research and a Professor of Internal Medicine and Pharmacology. Drs. Xie, Xiao, and Minna are members of the Harold C. Simmons Comprehensive Cancer Center.
“This shows the importance of multi-institutional collaborations such as LCMC to make such advances,” Dr. Minna said.
The work builds on a deep learning-based computational algorithm previously developed by Shidan Wang, Ph.D., Assistant Professor in the O’Donnell School of Public Health and lead author of the study. The algorithm, called Histology-based Digital Staining, can identify and classify multiple cell types in routine pathology images, Dr. Xie said.
Both Dr. Minna and Justin Bishop, M.D., Director of Anatomic Pathology and Professor of Pathology, provided critical oncology and pathology insights for the study, Drs. Xie and Xiao said.
The team’s integrated analysis included data from pathology images and genomic readouts, identifying potential molecular mechanisms of resistance to EGFR-targeted therapy, suggesting potential alternative treatment for TKI-resistant tumors, and providing a direction for future studies.
The study received support from National Institutes of Health/National Cancer Institute Cancer Center Support Grants [R35GM136375, U01AI156189, R01GM140012, R01GM141519, R01DE030656, U01CA249245, P50CA70907, P30CA142543, P30CA008748, P01CA129243], and the Cancer Prevention and Research Institute of Texas [RP190107 and RP180805].
Researchers from the University of Texas M.D. Anderson Cancer Center, Memorial Sloan Kettering Cancer Center, Vanderbilt University, Harvard, and the University of Colorado also participated in this work.
More information on this research, including the full list of authors and financial disclosures, can be found in the study.